MINT at W&V Summit - How the Integration of Autonomous Agents Promotes Governance, Efficiency, and Collaboration
Introduction
Aligned with our presentation at the 2025 W&V Summit in Munich, our latest article delves into the distinctions between traditional automation and the emerging agentic approach to Advertising Resource Management. It provides valuable insights into key factors advertisers should consider when upgrading their tech stack—from integration capabilities to ethical implications—helping them harness the full potential of this evolving technology.
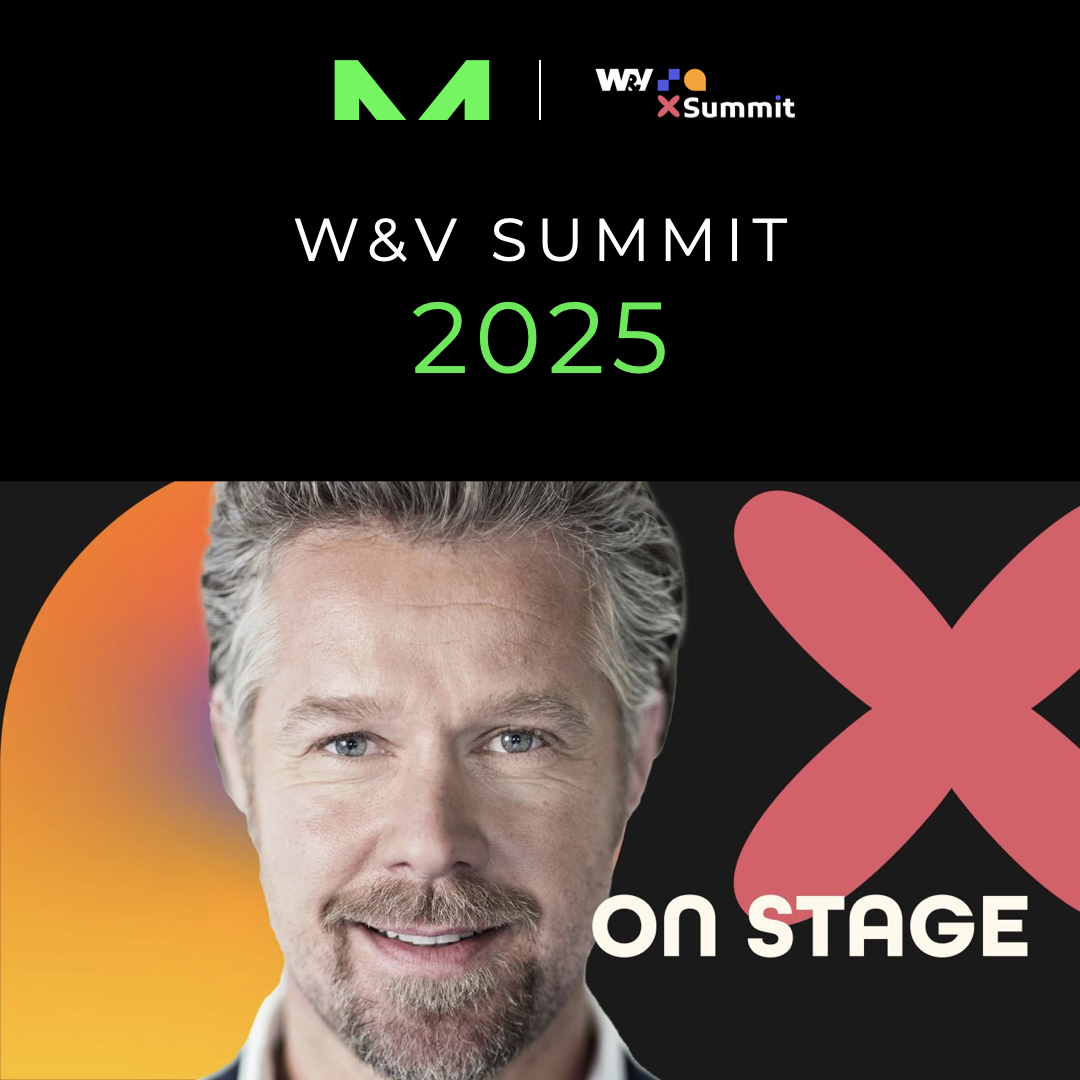
The Decision: AI Agents or Classic Automation?
The advertising landscape is undergoing a profound transformation with the advent of AI Agents. These advanced systems outperform traditional automation by handling complex tasks and making decisions based on extensive data analysis. So how do marketers choose whether they need powerful AI Agents or simpler rule-based automations like robotic process automation (RPA)?
The Power of Combination
The most effective advertising technology strategies often combine both approaches. Rules-based systems handle structured, repetitive tasks with clear decision criteria, while AI Agents master complex scenarios that require advanced memory, pattern recognition, and adaptive learning. By strategically employing each technology according to its strengths, advertisers can create synergistic systems that maximize both efficiency and effectiveness.
Resolving the AI Dilemma: Automation vs. Intelligent Agents
How AI Agents Work
The much-discussed AI Agents represent a paradigm shift from traditional approaches. Unlike their rule-based counterparts, these systems continuously learn from data, adapt to changing conditions and make autonomous decisions. They excel at recognizing subtle patterns, predicting outcomes and dynamically optimizing campaigns without constant human intervention.
The Role of Rule-based Automation
In contrast, rule-based automation works with explicit, predefined logic, i.e. it performs certain actions when predetermined conditions are met. This approach offers predictability, transparency and reliability for standardized processes.
Why Less is Sometimes More: Rule-based Automation
Ideal Use Cases for Automation
Rule-based automation supports many processes behind the scenes and ensures that campaigns run smoothly without the need for advanced AI. It works best when tasks:
- are repetitive and structured – e.g. automating reports or scheduling social media posts.
- are based on clear conditions – e.g. sending email sequences or pausing low-performing ads based on pre-set KPIs.
- do not rely on real-time data interpretation – rule-based automations do not “think”, they simply execute predefined actions.
Practical Example from Google Ads
A merchant using Google Ads, for example, can set up an automation to increase bids for products with a certain conversion rate and pause low-performing bids below a certain threshold. This doesn't require AI – just a well-thought-out and executed rule.
Unlock Potential: When to Implement a Team of AI Agents
The Superiority of AI in Complex Tasks
While automation follows predefined rules, AI Agents go one step further. They analyze, make predictions and make adjustments – for example, in the advertising process. Here they can perform complex analyses and make decisions in real time. AI Agents excel in scenarios where:
- Real-time decision making is required – AI can optimize ad bids in real time across multiple channels and dynamically allocate budgets based on performance.
- Personalization at scale is required – AI can generate targeted creatives that adapt to user behavior.
- Tasks require continuous learning – e.g. AI Agents can continuously develop in the context of ad fraud and recognize new patterns before advertisers are harmed.
Case Study: Media Buying with AI
An AI Agent managing the media buying of a global CPG brand, for example, can dynamically allocate ad spend across search, social, and programmatic channels based on live performance data. A rule-based system would not be able to adapt as quickly and would require manual intervention.
Performance as a Decision Criterion
In addition to the complexity of the task, performance is also a decisive criterion. Do the deployed automations not only solve the task satisfactorily, but also get the maximum performance out of it? Or is it conceivable that better results could be achieved with further, continuous adjustments? Are many rule adjustments or manual optimizations necessary? After all, granular improvements over a longer period of time could have a significant impact.
Revolutionizing Advertising with Multi-Agent Systems
From Tools to Partners
AI Agents are not just tools. They are partners that actively work with us. This shift from Single-Agent AI to Multi-Agent systems (MAS) is about to take hold in the advertising industry. An AI Agent is a piece of software that can reason, make decisions, and execute tasks independently. Multi-Agent systems work as a team, with individual AI agents collaborating on various aspects of a task.
The Advantages of Teamwork
Multi-Agent systems offer several advantages: AI Agents store, retrieve and share information dynamically, adapting to previous interactions. They can analyze data, detect errors and improve decision-making rather than blindly following instructions. Each Agent has specialized knowledge, similar to human experts, which allows them to specialize. Furthermore, AI Agents can be developed and replaced without disrupting the entire system, resulting in modularity and greater flexibility that makes it easier to adapt to change.
Various Architectural Models
Multi-Agent systems can be structured in different ways depending on the type of problem to be solved. “All-purpose” architectures resemble a network of experts, with each agent connected to the others and working together dynamically to solve unique problems quickly. Sequential architectures are more structured, with agents following a specific workflow and performing tasks one after the other, much like on an assembly line.
Optimizing Data for AI and Automation Success
The Importance of Data Management
Effective data management is critical for both rules-based automation and AI Agents. Rules-based automation relies on predefined rules and conditions that require accurate and consistent data inputs. For example, automating ad spending or triggering email sequences depends on reliable data about key performance metrics and user interactions.
Different Data Requirements
AI Agents, on the other hand, require significant amounts of data to learn, adapt, and make decisions. They benefit from an advanced “memory” in which they dynamically store, retrieve, and share information based on previous interactions.
Best Practices for Effective Data Management
To manage data effectively, advertisers should:
- Integrate data sources – Marketers should ensure that all relevant data from different platforms and systems is accessible to both rule-based automation and AI agents.
- Maintain data quality – Data must be regularly cleaned and updated to ensure accuracy and consistency.
- Leveraging data stores – Structured data stores that support retrieval augmented generation (RAG) enable AI to dynamically access the most relevant information.
The Future of the Advertising Industry: Hybrid Teams
The Technology Leap
The advertising industry is in the midst of a far-reaching technology leap. Before long, teams will naturally consist of human colleagues and AI Agents. When integrated into advertising ecosystems, AI agents drastically accelerate workflows while reducing manual intervention in repetitive processes. This “freedom” from operational activities in the advertising process saves time for what humans do best: strategic innovation and creative breakthroughs.
Transformation of Core Functions
The implications go well beyond operational efficiency. AI Agents are reimagining advertising's core functions – from campaign orchestration to media investment to customer engagement. Their enhanced “memory” allows them to store, access and share information in context, creating systems that learn from each interaction. Their analytical capabilities also enable them to process complex data sets, recognize hidden patterns, check themselves and generate insights that are not influenced by human prejudices.
Competitive Advantages of the Pioneers
Companies that use multi-agent frameworks and create intelligent ecosystems in which specialized AI components work together will gain a significant advantage. Forward-thinking brands and agencies won't just adopt these technologies. They'll use them as strategic accelerants to drive innovation, efficiency, and measurable results in an increasingly complex media environment. In this new paradigm, AI becomes more than just a tool—it becomes an indispensable partner in the ever-changing advertising landscape.
The Best of Both Worlds: How to Make the Right Decision
The choice between automation and AI Agents is not an either-or decision. Successful marketing teams use both technologies in a targeted manner for different tasks. Follow these recommendations:
- Analyze your processes – identify recurring, rule-based tasks for classic automation and complex, data-driven decisions for AI Agents.
- Start with simple automation – gain experience and then scale up to AI solutions where they offer real added value.
- Develop a data strategy – create the basis for both technologies with clean, integrated data sources.
- Think in ecosystems – plan for the long term with multi-agent systems that combine different specializations.
- Form hybrid teams – prepare your employees to work with AI Agents and focus on strategic tasks.
The intelligent combination of both technologies will become the decisive competitive advantage. Those who set the right course today will optimally position their company for the AI-driven future of marketing.
The original article was published on W&V and it is available at this link.